What are Recommender Systems?
A recommendation system, also called a recommender system, is a software solution that makes personalized suggestions or recommendations to users for products, services, or content. It is based on the user’s preferences, behavior and interactions. Such systems are often used in online stores, streaming platforms, social networks, but also on modern learning platforms like chunkx. On learning platforms, they are only usually limited to recommending new courses to learners from the respective platform or from a limited number of partners. We think this can be done better!
Recommender Systems in chunkx
In chunkx, we not only process which courses a learner is taking, but we can directly analyze the content of the many individual learning units. This allows us to make comparisons in terms of content. For example, if you learn a lot about diverse leadership, we can use the content of your learning units and your learning data to match them with skills and generate appropriate recommendations:
1) Matching courses in chunkx
Okay, that’s not a surprise yet, but it’s a very important feature for our customers who use our micro-learning platform.
2) Suitable courses outside of chunkx
Now we are getting closer to innovation. Using AI and well-placed data extraction, we can find suitable courses for you to continue learning. Skills, development goals and other parameters can also be taken into account.
3) Articles, studies and updates
chunkx already supports you intensively in the transfer of learning. But with our recommender system, we also make it easy for you to benefit from new articles, studies, and general updates on your topics. Let’s stay with the example of Diverse Leadership: If you have a great course with a subsequent learning subscription in chunkx, you don’t want to stop at this level of content, but rather continue to develop. As long as you keep the subscription to the channel, we will automatically compare your learning data and skills with newly found items and let you know as soon as we find something suitable for you! In this way, we support you not only in the transfer of learning, but also in continuous learning.
Recommendation sources
Quality is a core requirement for our automatically generated recommendations. The first step towards this is to control the sources from which new recommendations may be generated in principle. There are different levels here:
- Selected sources
Together with our customers, we determine which sources they want us to crawl on a regular basis. This ensures that only sources requested by the customer are considered for new recommendations. Crawling means that a robot looks at the website again and again and analyzes what new has been published. This content is vectorized to make it easier to compare with learning data. - In-house sources
Of course, customers have even more control over their internal data. And these can also be used for recommendations: Similar to the approach above, we vector internal data, e.g., that of a product database or knowledge platform, and respond to identified knowledge gaps in learners with the perfect recommendations on internal articles. - The free internet
Especially for topics that are highly topical, such as Generative AI, and where things can change on a daily basis, we recommend that customers refrain from restricting sources. Without this restriction, we can find even more suitable recommendations for courses, articles and updates.
Validation of recommendations
Especially with innovative automation, it is important to set up the validation of the results properly. Depending on the use case, an initial validation is already ensured via the restriction and selection of sources. Since our recommender system is primarily based on content proximity between learning units and new courses or articles, there is basically a very good chance of a good fit. All results that are good enough from this point of view end up on a shortlist.
We then have the shortlist analyzed with GPT-4 and compared again with the baseline situation, i.e. the learner data. We only use services via Microsoft Azure here, so we can ensure that none of OpenAI’s data may be used outside of our specific purpose and that all data is processed in Europe (specifically, in France). After processing, a few top results remain.
The top results are humanly checked again before publication, so that really nothing can go wrong. This is true for our asynchronous recommendations, but not for adhoc recommendations, for example, when a learner wants a new course recommendation right now.
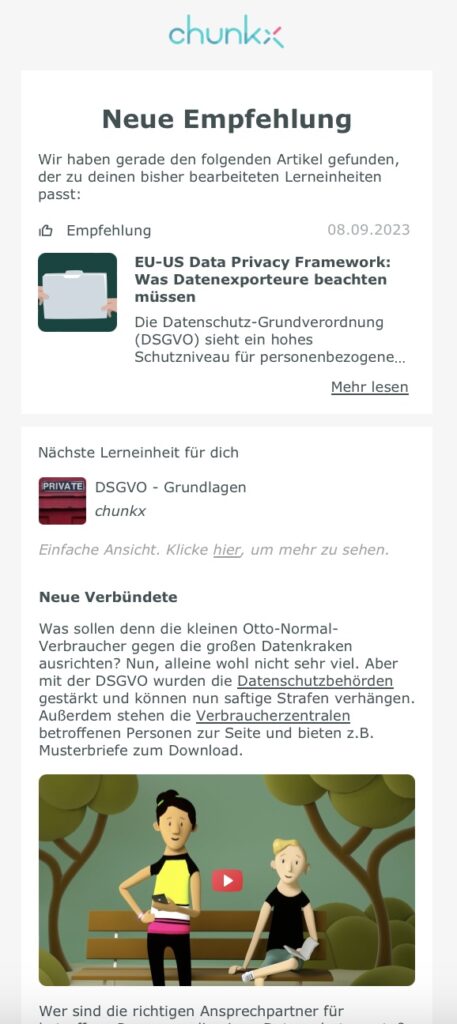
Linking with next learning unit
In the image above you can see an example of a recommendation sent by email. What you see below the recommendation: every communication event is used by chunkx to send learners the next learning unit. In this way, we support continuous learning and enable learning directly in the flow-of-work in the channels that people already use anyway (currently we support email and MS Teams).
When do you start with chunkx?
With chunkx, we’ve created the solution to turn your one-off learning activities into continuous learning experiences, including through our recommender systems. Talk to us about your learning culture and the changes in your organization and how chunkx can best support you.
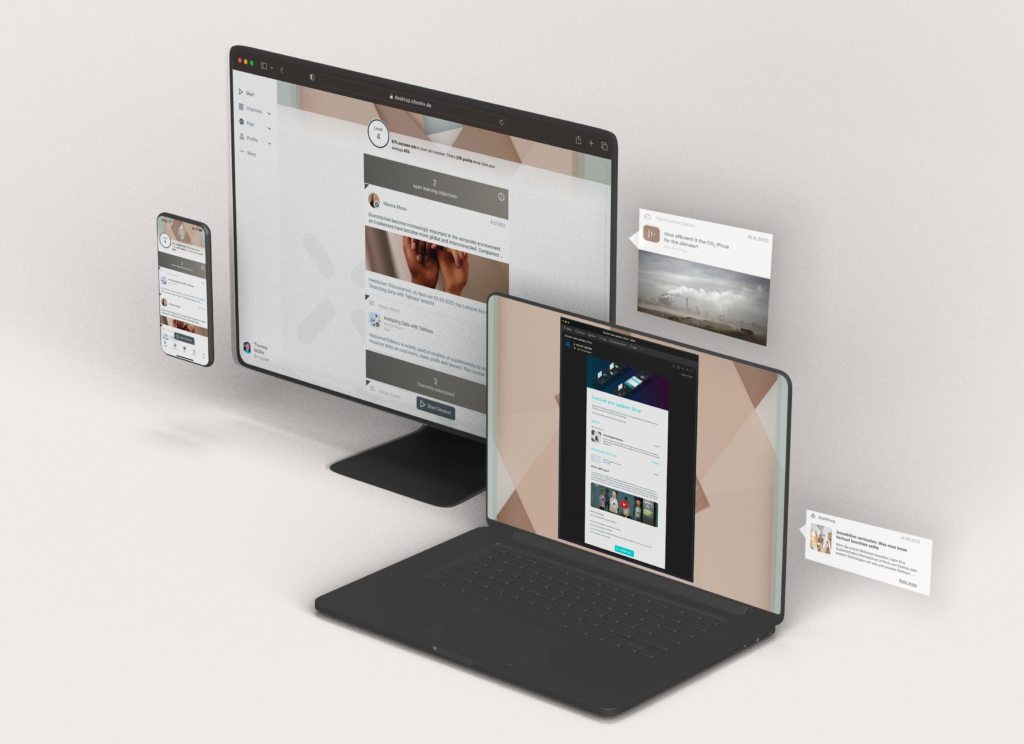